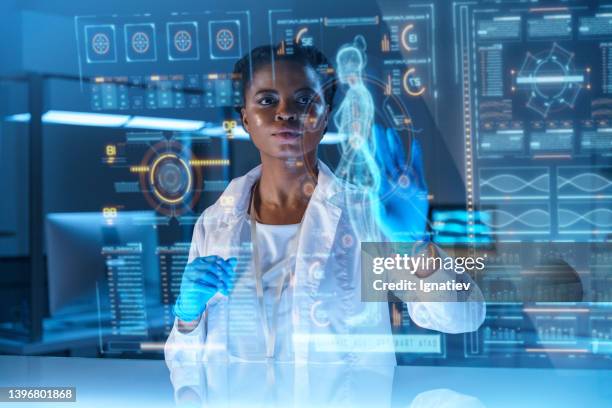
A team from The Lancet Digital Health released research about artificial intelligence (AI) performance during breast cancer screening within the mammography screening with artificial intelligence (MASAI) trial. Research data indicates that artificial intelligence technology enhances cancer detection capabilities and cuts down radiologist screening tasks. The study has brought uncertainty regarding both its ability to produce false positive results and its effectiveness in detecting relevant cancers.
Why AI in Mammography Screening?
Breast cancer exhibits different levels of complexity as it covers both indolent and high-aggressiveness forms. Early detection and treatment preparation programs heavily depend on screening measures. Both radiologists examine mammography images to validate diagnoses in European medical screening programs today.
By using AI tools radiologists can experience less workload but simultaneously achieve better cancer detection rates. Knowledge from earlier research indicates AI technology has the potential to boost cancer detection capabilities but medical experts still wonder if AI systems might produce errors by reporting non-existent cancers or focusing mainly on harmless growths.
Inside the MASAI Trial
The MASAI trial evaluated mammography screening that incorporated AI assistance against the established method of double reading. Female participants in this study come from Sweden’s national mammography screening program. The Transparent AI system reviewed mammograms and generated risk scores ranging from one to ten in the AI-supported team. Today double reading occurred only for examinations receiving a score of 10 while assessments with scores 1-7 and 8-9 only required a single review.
The Transpara system alerted healthcare personnel to treat the 1% most risky cases as urgent cases for added medical scrutiny. The test group performed double reading as per their routine procedures without using AI systems. The research explored tumor attributes together with lymph node condition and metastasis spread but also investigated AI contributions toward identifying cancer cases and screening repetition frequency and interpreter workload demands.
Findings: AI Increases Breast Cancer Detection, But at What Cost?
The research enrolled 53,043 participants under the AI group while the control group consisted of 52,872 participants. AI detection algorithms identified 3,800 cases as high risk with 655 extra-high risk cases. An unspecified number of 368 patients did not obtain AI risk scores.
Key Findings:
- The combination of artificial intelligence with double reading methods increased the identification of Breast cancer cases by 29% above conventional screening procedures.
- The analysis revealed modest improvements in false-positive rates together with slight increases in recall rates while positive predictive value (PPV) for recall was the only metric that demonstrated enhancement.
- Through its implementation, AI cut the total workload for screening by 44% which translated to reduced screen readings of 48,444 while consensus meeting numbers increased by 65 sessions.
In the AI-supported group:
A higher number of 941 participants underwent recall examinations for findings identified in their mammograms than in the control group which had 847 patients. Symptoms led to the recall of 169 participants while control participants needed recalls of 180 participants.
The frequency of Breast cancer detection in all age brackets grew while false-positive reports began to increase starting from age sixty onward.
The AI-supported screening enabled an extra discovery of 76 cancers
- 23 more in situ cancers
- 53 more invasive cancers
AI technology discovered additional cancers among all histological types with a distinct emphasis on invasive cancers of no special category. AI screening revealed more cases of cancers at all histological grades where Grade I tumors experienced the greatest rise in identification.
- Pneumococcal vaccinations resulted in 29 luminal A (less aggressive) and 21 non-luminal A invasive cancer discoveries.
- The AI-supported group revealed an additional 46 cases of node-negative cancer and 5 cases of node-positive cancer.
- The amounts of stage 2+ TNM cases remained equivalent between the two experimental groups.
Most cases of non-luminal cancer discovered through AI testing were determined to have no node involvement which questions their clinical importance.